Shobha Vasudevan (Google)
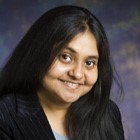
Title: Learning Semantic Representations of Hardware Designs for Verification and Test
Abstract
In this talk, I will present our research in applying ML for chip design verification, conducted in Google Brain/DeepMind in collaboration with our TPU design teams. This was the first technique that used deep learning to solve highly computationally complex problems of design verification (NeurIPS 2021). The technique showed order of magnitude benefits as compared to the state-of-the-art tools in that space and manual verification used by the chip design teams. It has also been productionized and applied within Google's TPU chip design process. Extensions of the work to debugging, monitoring, and synthesis have also been used in TPU design.
Bio
Shobha Vasudevan is an AI/ML researcher and technologist in Google. She currently leads ML performance optimization tools and algorithms for datacenters and cloud in Google.
Prior to joining the performance team, she was in Google DeepMind and Google Brain (2019-2022), where she led the research and development of deep learning based solutions for chip design and compiler optimizations. The products of this research collaboration have been productionized in Googleś TPU chips and XLA compiler. Prior to joining Google, she was a tenured associate professor in the ECE/CS departments of the University of Illinois at Urbana-Champaign (2009-2017). Shobha received her PhD in ECE from the University of Texas at Austin in 2009. Shobhaś technology and research expertise is in building scalable automation solutions for various problems across the ML stack, ranging from model level, to compiler and hardware level. She has invented and built solutions for performance, reliability, testing, verification across the generative AI vertical stack. Shobha is also actively involved in technical program committees, journal editorships, NSF panels, and runs a program within Google for mentoring women in STEM.